Quantitative analysis of endometrial hyperplasia
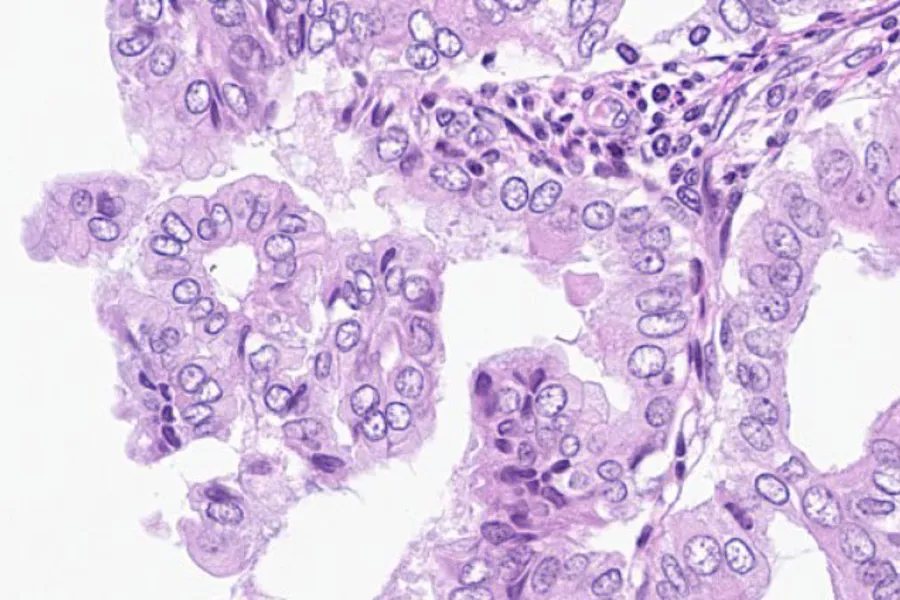
Endometrial hyperplasia is a condition defined by abnormal proliferation of glands in the lining of the uterus. Endometrial hyperplasia without atypia is unlikely to progress whereas endometrial atypical hyperplasia has a high-risk of progression to cancer. Current classification schemes have poor reproducibility, which limits their prognostic capability.
So far in this work package we have developed an automated and objective machine-learning based tool for the prognostic assessment of endometrial hyperplasia, to reduce risk of over- and under-treatment of patients.
The algorithm was built using the Visiopharm® platform and combined with a logistic regression model to create the ENDOAPP. The ENDOAPP measures the features of percentage stroma and nuclear variation in the epithelial glands of the suspect lesion. These features are used to output a risk score indicating the likelihood of progression to endometrial cancer. The model is now in the process of being validated regionally, and a large multi-centre validation study is planned.
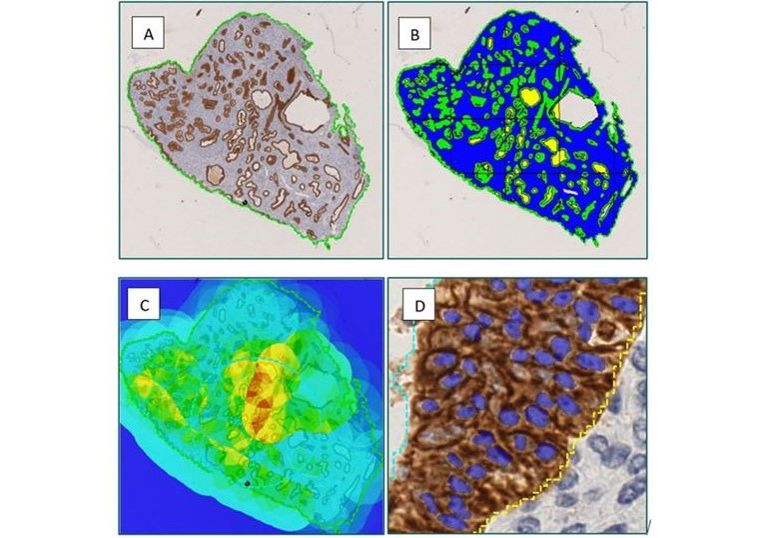
The figure above shows the APP workflow for risk classification of endometrial hyperplasia. A) Detection of endometrial tissue B) Labelling of endometrial structures including epithelial glands (green), lumen (yellow) and surrounding stroma (blue) C) Heatmap to identify the most dense region of glands D) Labelling of gland nuclei.